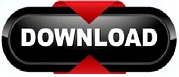
The advantages of these new technologies are manyfold. The most popular and highest throughput ST technologies such as the 10X Visium ( 1) and slide-seq ( 2, 6) technologies, relies on spots (probe capture areas), rather than on fluorescent imaging, which still today remains lower throughput ( 3, 7). ST allows for localized measurements of RNA content, mapped directly in the interrogated tissues. These have spawned a subfield of transcriptomics coined spatial transcriptomics (ST), also known as spatially resolved transcriptomics( 4, 5). Recently, technologies capturing the in-situ diversities of the transcriptomic landscape have emerged ( 1–3). Furthermore, AntiSplodge demonstrates top of the line performance when compared to current state-of-the-art tools. In particular, for the hippocampus from where the cells originate. We further deconvolute the mouse brain, where spot patterns correctly follow that of the underlying tissue. To demonstrate AntiSplodge, we deconvolute the human heart and verify correctness across time points. AntiSplodge is designed to be easy, fast and intuitive while still being lightweight. Therefore, we present AntiSplodge, a simple feed-forward neural-network-based pipeline designed to effective deconvolute ST profiles by utilizing synthetic ST profiles derived from real-life SC datasets. Yet, most existing tools are cumbersome, and we found them hard to integrate and properly utilize. This is usually done by incorporating metrics from single-cell (SC) RNA, from similar tissues. Recently, applications have been proposed to tackle the deconvolution process, to gain knowledge about which cell types (SC) are found within. However, with the current technologies, measurements consist of mRNA transcript profiles of mixed origin. With the current surge of spatial transcriptomics (ST) studies, researchers are exploring the deep interactive cell-play directly in tissues, in situ.
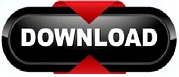